GeoJSON is a structured format for encoding a variety of geographic data structures like land topology, governmental boundaries, etc. It has structures for points, lines, polygons, and discontiguous collections of all of them. GeoJSON has been in use since the late 2000s.
TopoJSON is a more recent extension to GeoJSON, which brought a key innovation: when encoding the boundary between two regions, both regions contain a representation of that boundary. Using TopoJSON frequently results in much smaller files by separating out the definition of arcs from the collections of those arcs, allowing adjacent regions to both reference the same data describing the border between them. It also adds delta-encoding where points are encoded as a delta from the previous point, which tends to result in smaller numbers for dense topographical areas. TopoJSON was added as part of D3.js, an extremely popular 3d visualization JavaScript library, and has thus spread rapidly.
Today's topic is creation of customized TopoJSON files for various purposes. Mike Bostock, one of the creators of D3, wrote a series of articles about command line tools available for working with cartographic data. I wanted to develop a visualization of climate model results for regions of the world like Latin America or the Middle East and Africa, and found these articles immensely helpful in creating a TopoJSON file to support this. Part 3, which introduces TopoJSON and the CLI tools to work with it, was especially helpful.
Overview
The overall process we'll cover today is:
- Annotate existing geometries in a TopoJSON file with a new grouping name.
- Merge the annotated geometries to create new groupings.
- Remove the original geometries and supporting topology data.
- Profit!
We'll step through this series of commands:
cat world-countries.json |\ python region_annotate.py |\ topomerge regions=countries1 -k "d.region" |\ topomerge countries1=countries1 -f "false" | toposimplify \ > world_topo_regions.json
(Step 1) Annotate existing regions: region_annotate.py
We start from world-countries.json provided by David Eldersveld under an MIT license. The file defines geometry for each country by name and country code:
"countries1": { "type": "GeometryCollection", "geometries": [ { "arcs": [...etc... ], "type": "MultiPolygon", "properties": { "name": "Argentina", "Alpha-2": "AR" }, "id": "ARG" },
In Python, we create a tool with a mapping table of country names to the regions we want to define:
region_mapping = { "Albania": "Eastern Europe", "Algeria": "Middle East and Africa", "Argentina": "Latin America",
We read in the JSON, iterate over each country, and add a field for the region it is supposed to be in:
d = json.load(sys.stdin) for country in d['objects']['countries1']['geometries']: name = country['properties']['name'] region = region_mapping[name] country['region'] = region json.dump(obj=d, fp=sys.stdout, indent=4)
If one were to examine the JSON at this moment, there would be a new field:
"countries1": {
"type": "GeometryCollection",
"geometries": [
{
"arcs": [...etc...
],
"type": "MultiPolygon",
"properties": {
"name": "Argentina",
"Alpha-2": "AR"
},
"id": "ARG",
"region": "Latin America"
},
(Step 2) Merge annotated regions: topomerge
topomerge is part of the topojson-client package of tools, and exists to manipulate geometries in TopoJSON files. We invoke TopoJSON to create new geometries using the field we just added.
topomerge regions=countries1 -k "d.region"
The "regions=countries1" argument means to use the source object "countries1" and to target a new "regions" object. The -k argument defines a key to use in creating the target objects, where d is the name of each source object being examined. We're tell it to use the 'region' field we added in step 1.
If we were to examine the JSON at this moment, the original "countries1" collection of objects would be present as well as a new "regions" collection of objects.
"objects": {
"countries1": {
"type": "GeometryCollection",
"geometries": [
{
"arcs": [...etc...
],
"type": "MultiPolygon",
"properties": {
"name": "Argentina",
"Alpha-2": "AR"
},
"id": "ARG",
"region": "Latin America"
},
...etc, etc...
"regions": {
"type": "GeometryCollection",
"geometries": [
{
"type": "MultiPolygon",
"arcs": [...etc...
],
"id": "Latin America"
},
(Step 3) Remove original regions
As we don't use the individual countries in this application, only regions, we can make the file smaller and the UI more responsive by removing the unneeded geometries. We use topomerge to remove the "countries1" objects:
topomerge countries1=countries1 -f "false"
As before, the "countries1=countries1" argument means to use the source object "countries1", and to target the same "countries1" object. We're overwriting it. The -f argument is a filter, which takes a limited JavaScript syntax to examine each object to determine whether to keep it. In our case we're removing all of the objects unconditionally, so we pass in false.
If we were to examine the JSON at this moment, we would see an empty "countries1" collection followed by the "regions" collection we created earlier.
"objects": {
"countries1": {
"type": "GeometryCollection",
"geometries": []
},
"regions": {
"type": "GeometryCollection",
"geometries": [
{
"type": "MultiPolygon",
"arcs": [...etc...
],
"id": "Latin America"
},
However we're not quite done, as the arcs which define the geometry between all of those countries are still in the file, though not referenced by any object. We use toposimplify, part of the topojson-simplify package of tools, to remove the unreferenced arcs.
topomerge countries1=countries1 -f "false" | toposimplify
(Step 4) Profit!
That's it. We have a new TopoJSON file defining our regions. Rendered to PNG:
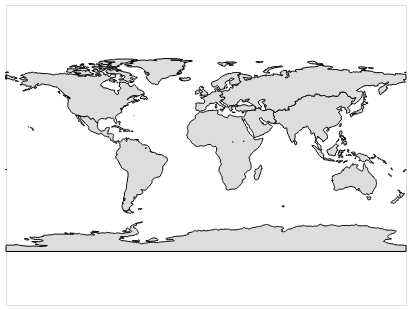
The JSON file viewed using github's gist viewer requires a bit of explanation: the country boundaries seen here are being rendered by github from OpenStreetMap data. The country boundaries are not present in the JSON file we created, only the regional boundaries as seen in the PNG file.